by Marcus Munafò @MarcusMunafo
This blog originally appeared on the Mental Elf site on 11th Febraury 2016.
Understanding who responds well to treatment for depression is important both scientifically (to help develop better treatments) and clinically (to more efficiently prescribe effective treatments to individuals). Many attempts to predict treatment outcomes have focused on mechanistic pathways (e.g., genetic and brain imaging measures). However, these may not be particularly useful clinically, where such measures are typically not available to clinicians making treatment decisions. A better alternative might be to use routinely- or readily-collected behavioural and self-report data, such as demographic variables and symptom scores.
Chekroud and colleagues (2015) report the results of a machine learning approach to predicting treatment outcome in depression, using clinical (rather than mechanistic) predictors. Since there are potentially a very large number of predictors, examining all possible predictors in an unbiased manner (sometimes called “data mining”) is most likely to produce a powerful prediction algorithm.
Machine learning approaches are well suited to this approach, because they can identify patterns of information in data, rather than focusing on individual predictors. They can therefore identify the combination of variables that most strongly predict the outcome. However, prediction algorithms generated in this way need to be independently validated. By definition, they will predict the outcome in the data set used to generate the algorithm (the discovery sample). The real test is whether they also predict similar outcomes in independent data sets (the replication sample). This avoids circularity, and increases the likelihood the algorithm will be clinically useful.
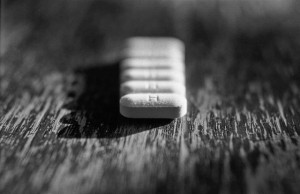
Clinicians currently have no empirically validated mechanisms to assess whether a patient with depression will respond to a specific antidepressant.
Methods
The authors used data from a large, multicenter clinical trial of major depressive disorder (the STAR*D trial – Trivedi et al, 2006) as their discovery sample, and a separate clinical trial (the CO-MED trial, Rush et al, 2011) as their replication sample. Data were available on 1,949 participants in the STAR*D trial, and 425 participants in the CO-MED trial. The CO-MED trial consisted of three treatment groups, with participants randomised to receive either:
- Escitalopram-placebo
- Bupropion-escitalopram
- Venlafaxine-mirtazapine
The authors built a predictive model using all readily-available sources of information that overlapped for participants in both trials. This included:
- A range of sociodemographic measures
- DSM-IV diagnostic items
- Symptom severity checklists
- Eating disorder diagnoses
- Whether the participants had taken specific antidepressant drugs
- History of major depression
- The first 100 items of the psychiatric diagnostic symptoms questionnaire.
In total, 164 variables were used.
For the training process, the machine learning approach divided the original sample (using the STAR*D data) into ten subsets, using nine of those in the training process to make predictions about the remaining subset. This process was repeated ten times, and the results averaged across these repeats. The final model built using the STAR*D data was then used to predict outcomes in the each of the CO-MED trial treatment groups separately.
The model was developed to detect people for whom citalopram (given to everyone in the first 12 weeks of the STAR*D trial) is beneficial, rather than predicting non-responders. It was constrained to require only 25 predictive features (i.e., clinical measures), to balance model performance (which should be greater with an increasing number of predictors) with clinical usability (since an algorithm requiring a very large number of predictors may be difficult to implement in practice).
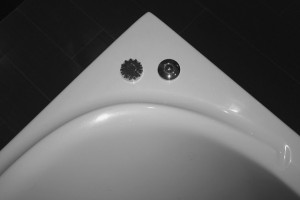
Only 11-30% of patients with depression reach remission with initial treatment, even after 8-12 months.
Results
The top three predictors of non-remission were:
- Baseline depression severity
- Feeling restless during the past 7 days
- Reduced energy level during the past 7 days
The top three predictors of remission were:
- Currently being employed
- Total years of education
- Loss of insight into one’s depressive condition
Overall, the model predicted outcome in the STAR*D data with:
- An accuracy of 64.6% – it identified 62.8% of participants who eventually reached remission (i.e., sensitivity), and 66.2% of non-remitters (i.e., specificity)
- This is equivalent to a positive predictive value (PPV) of 64.0% and a negative predictive value (NPV) of 65.3%
- The performance of the model was considerably better than chance (P = 9.8 × 10-33)
In the CO-MED data, the model:
- Pedicted outcome in the escitalopram-placebo group:
- Accuracy 59.6%, 95% CI 51.3% to 67.5%,
- P = 0.043,
- PPV 65.0%,
- NPV 56.0%.
- Escitalopram-bupropion group
- Accuracy 59.7%, 95% CI 50.9% to 68.1%,
- P = 0.023,
- PPV 59.7%,
- NPV 59.7%.
However, there was no statistical evidence that it performed better than chance in the venlafaxine-mirtazapine group:
- Accuracy 51.4%, 95% CI 42.8% to 60.0%,
- P = 0.53,
- PPV 53.9%,
- NPV 50.0%.
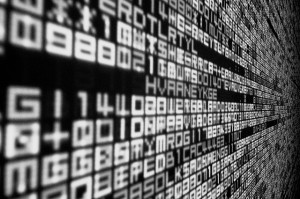
Could predictive models that mine existing trial data help us prospectively identify people with depression who are likely to respond to a specific antidepressant?
Conclusions
The authors conclude that their model performs comparably to the best biomarker currently available (an EEG-based index) but is less expensive and easier to implement.
The outcome (clinical remission, based on a final score of 5 or less on the 16-item self-report Quick Inventory of Depressive Symptomatology, after at least 12 weeks) is associated with better function and better prognosis than response without remission.
Strengths and limitations
There are some strengths to this study:
- First, it attempts to build a prediction algorithm using data that are already collected routinely in clinical practice, or could be easily incorporated into routine practice.
- Second, the prediction algorithm shows some evidence of generalisability to an independent sample.
- Third, the algorithm also shows some degree of specificity, by performing best in the escitalopram-treated groups in the CO-MED data.
However, there are also some limitations:
- First, there is a clear reduction in how well the algorithm predicts treatment outcome in the discovery sample (STAR*D) compared with the replication sample (CO-MED). This illustrates the need for an independent replication sample in studies of this kind.
- Second, and more importantly, although the algorithm performed better in the escitalopram-treated groups in CO-MED, it’s not clear that there was any evidence that performance was different across the three arms – the 95% confidence intervals for the venlafaxine-mirtazapine group (42.8% to 60.0%) include the point estimates for the other two groups (escitalopram-placebo: 59.6%, escitalopram-bupropion: 59.7%). Therefore, although there is some evidence of specificity, it is indirect, and the algorithm may in fact predict treatment outcome in general, rather than in those who have received a specific treatment, at least in part.
- Third, models of this kind cannot tell us whether the variables that predict treatment outcome are causal. This may not matter if our focus is on clinical prediction, although if they are not causal then the prediction algorithm may not generalize well to other populations. For example, in both the discovery and replication sample participants had been recruited into clinical trials, and therefore may not be representative of the wider population of people with major depressive disorder. Causal anchors are likely to be more important if we are interested in mechanistic (rather than clinical) predictors.
Summary
Ultimately, being able to simultaneously identify individuals likely to respond well to drug A and not respond to drug B will be clinically valuable, and is the goal of stratified medicine. This study represents only the first step towards being able to identify likely responders and non-responders for a single drug (in this case, citalopram); in particular, although there was some evidence for specificity in this study, it was relatively weak.
Ultimately, with larger datasets that include multiple treatment options (including non-pharmacological interventions), it may be possible to match people to the treatment option they are most likely to respond successfully to. The focus on routinely- or readily-collected data means that it gives an insight into what clinical prediction algorithms for treatment response in psychiatry may look like in the future.
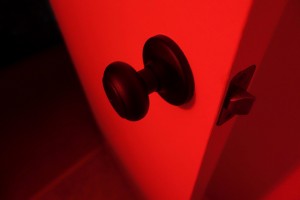
This innovative study (and others like it) may open the door to predict more personalised medicine for people with depression.
Links
Primary paper
Chekroud AM, Zotti RJ, Shezhad Z, Gueorguieva R, Johnson MK, Trivedi MH, Cannon TD, Krystal JH, Corlett PR. (2015) Cross-trial prediction of treatment outcome in depression: a machine learning approach. Lancet Psychiatry 2015. doi: S2215-0366(15)00471-X [Abstract]
Other references
Trivedi MH, Rush AJ, Wisniewski SR, Nierenberg AA, Warden D, Ritz L, Norquist G, Howland RH, Lebowitz B, McGrath PJ, Shores-Wilson K, Biggs MM, Balasubramani GK, Fava M; STAR*D Study Team. (2006) Evaluation of outcomes with citalopram for depression using measurement-based care in STAR*D: implications for clinical practice. Am J Psychiatry. 2006 Jan;163(1):28-40. [PubMed abstract] [Wikipedia page]
Rush AJ, Trivedi MH, Stewart JW, Nierenberg AA, Fava M, Kurian BT, Warden D, Morris DW, Luther JF, Husain MM, Cook IA, Shelton RC, Lesser IM, Kornstein SG, Wisniewski SR. (2011) Combining medications to enhance depression outcomes (CO-MED): acute and long-term outcomes of a single-blind randomized study.Am J Psychiatry. 2011 Jul;168(7):689-701. doi: 10.1176/appi.ajp.2011.10111645. Epub 2011 May 2. [PubMed abstract]
Photo credits
- r2hox CC BY 2.0
- r. nial bradshaw CC BY 2.0
- Edgar Sousa CC BY 2.0
- r2hox CC BY 2.0
- Dean Hochman CC BY 2.0
– See more at: http://www.nationalelfservice.net/mental-health/depression/can-a-machine-learning-approach-help-us-predict-what-specific-treatments-work-best-for-individuals-with-depression/#sthash.wvpEojY8.dpuf